Appendix B: Future Workforce of Consumer Modelling Approach: Packaged Goods and Retail Industries in the 1. Constructed consistent data that links automation US: Econometric Model, 2017 probabilities, skills, job activities, job descriptions and The model is used to identify the portion of employment employment data subject to automation under different assumptions of the 2. Defined those job activities that require strong human- “state of skills” in the workforce. It employs an estimated like skills (that are not automatable) through regression inverse relationship between the importance of skills that run analysis with the machine and time allocation to “human-like” tasks 3. Computed the share of employment in each country within occupations, on the one hand, and the probability of that is subject to total automation (= more than 75% automation, on the other. The analysis takes a task-based probability), based on econometrics to estimate how the approach that accounts for human versus machine-like skills automation probability depends on PIACC activities of and tasks in occupations, in a similar way to the OECD’s employees 52 research by Arntz, Gregory and Zierahn (2016), to address an important gap in occupation-based estimations (e.g. Frey 4. Computed the average automation probability of each 53 task grouping to calculate the total amount of tasks that and Osborne, 2013), which assumes that occupations as a whole, rather than single tasks, can be automated by can be automated across all jobs technology. This leads to an overestimation of automation – in reality, most occupations contain a substantial share of tasks that are hard to automate. This analysis is based on the state of technologies today and accounts for future technological improvement (advancement/adoption) in a constant (linear) fashion. It considers the fact that the adoption of new technologies is often a slow process and workers are able to adjust by learning skills and switching tasks, preventing unemployment. The share of workforce subject to automation must not be equated to its current “as of today” possibilities. Assumptions were made about the pace of reskilling the workforce in the future in terms of “run with the machine” skills, the reallocation of working time to human-like tasks and the reduction in learning years needed by the workforce in the United States to be ready to “run with the machine”. Methodology: – Country-specific analysis for CPG and retail industries in the United States – Data basis: O*NET database, OECD PIACC survey, the work of C.B. Frey and M.A. Osborne (Oxford University), combined with the work supply demographics of the individual countries derived from the respective national account statistics on employment 26 Operating Models for the Future of Consumption
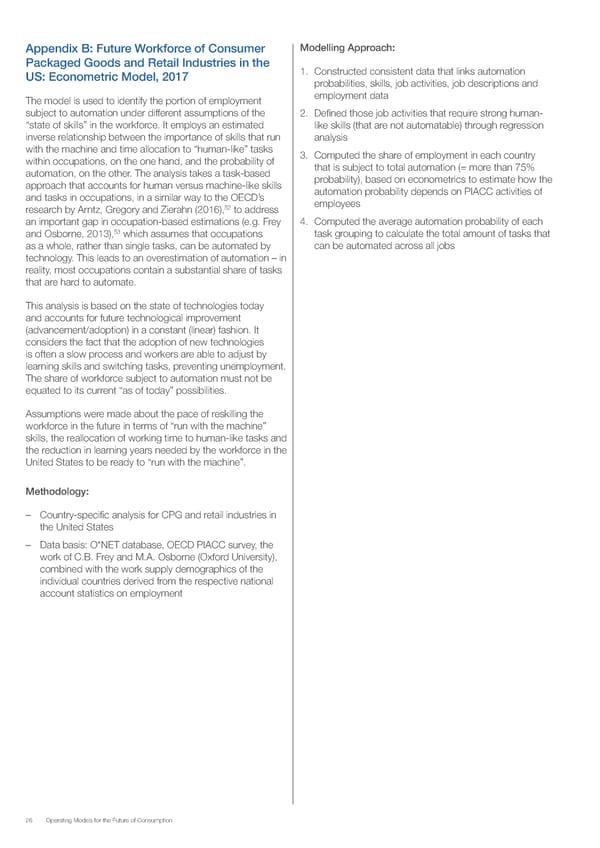